MLOPS Training in Hyderabad
- Real-Time Projects
- Internship Till You Placed
- One-One Mentorship
MLops Training in Hyderabad
Batch Details
Trainer Name | Madhumathi, Dr. Prasad |
Trainer Experience | 10+ Years, 20+ Years |
Next Batch Date | 21st July 2025 (09 : 00 AM IST Offline) 14th July 2025 (08: 00 AM Online) |
Training Modes | Classroom Training (Hyderabad), Online Training |
Course Duration | 3 Months |
Call us at | +91 9885044555 |
Email Us at | genaimasters@gmail.com |
Demo Class Details | Click Here to Chat on Whatsapp |
MLops Training in Hyderabad
Course Curriculum
What is MLOps?
- MLOps stands for Machine Learning Operations.
- It combines Machine Learning, DevOps, and Data Engineering.
- Helps manage, deploy, and monitor ML models effectively in production.
- Ensures automation, collaboration, and faster delivery of ML solutions.
Different Stages in MLOps
- Data Collection – Gathering and preparing data for training.
- Model Training – Creating and testing ML models.
- Model Versioning – Tracking different versions of models and data.
- Model Deployment – Making models available to users.
- Monitoring – Checking performance and identifying issues in real-time.
- Model Retraining – Updating models based on new data or performance drops.
ML Project Lifecycle
- Define the Problem – Understand the business goal.
- Collect & Prepare Data – Clean and process data for training.
- Build the Model – Train and tune machine learning models.
- Evaluate the Model – Test accuracy and reliability.
- Deploy the Model – Launch the model in a real-world environment.
- Monitor & Improve – Track performance and make updates as needed.
Job Roles in MLOps
- MLOps Engineer – Builds and manages ML pipelines and infrastructure.
- ML Engineer – Focuses on model development and optimization.
- Data Engineer – Handles data pipelines and storage systems.
- DevOps Engineer (ML) – Maintains deployment and automation systems.
- AI/ML Architect – Designs the end-to-end machine learning infrastructure.
What is the Development Stage of an ML Workflow?
- This is where data scientists build, train, and test models.
- Focuses on exploring data, creating features, and evaluating models.
- Typically involves experimentation and iteration to find the best model.
Pipelines and Steps
- A pipeline is a sequence of steps that process data and train models.
- Common steps include
- Data loading
- Preprocessing
- Training
- Evaluation
- Deployment prep
- Pipelines make the workflow repeatable and scalable.
Artifacts
- Artifacts are the outputs of each pipeline step.
- Examples include:
- Processed datasets
- Trained models
- Evaluation metrics
- They help track progress and reuse results.
Materializers
- Materializers handle how artifacts are saved and retrieved.
- They help in storing outputs in a way that can be reused later.
- Example: Saving a trained model as a .pkl file.
Parameters & Settings
- Parameters are variables used in training (e.g., learning rate, batch size).
- Settings configure how pipeline steps are run (e.g., number of retries, caching).
Changing parameters allows for easy experimentation without editing code.
Stacks & Components
- A stack is the complete setup or environment used to run an ML workflow.
- It includes different components like:
- Orchestrator (controls workflow steps)
- Artifact store (saves data and models)
- Container or runtime (where the code runs)
Orchestrators
- Orchestrators manage and schedule pipeline runs.
- Ensure tasks run in the right order with dependencies handled.
- Popular tools: Airflow, Kubeflow, Prefect, ZenML Orchestrator.
Artifact Stores
- These are storage locations where artifacts (datasets, models, logs) are saved.
- Can use cloud (e.g., S3, GCS) or local disk.
- Helps in reproducibility and version tracking.
Flavors
- Flavors define the specific tool or technology used for each component.
- Example:
- Orchestrator flavor: Apache Airflow
- Artifact store flavor: AWS S3
- Model deployer flavor: MLflow
This makes stacks modular and customizable.
ML Server Infrastructure
- Refers to the hardware and cloud setup used to run ML models.
- Can be on-premise servers or cloud platforms like AWS, GCP, or Azure.
- Needs to support scalability, high availability, and performance.
- Server Deployment
- Deploying ML models on servers so they can serve predictions.
- Common methods: REST APIs, batch jobs, streaming.
- Tools: Docker, Kubernetes, Flask, FastAPI, TensorFlow Serving.
Metadata Tracking
- Automatically stores information about:
- Model versions
- Dataset versions
- Training parameters
- Results and logs
- Helps in debugging, auditing, and reproducibility.
Collaborations
- Enables teamwork across data scientists, ML engineers, and DevOps.
- Involves shared pipelines, access control, version control (Git), and project tracking.
- Promotes faster and more organized development.
Dashboards
- Visual tools to monitor:
- Model performance
- Data drift
- Training metrics
- System health
- Tools: Grafana, Prometheus, MLflow UI, Streamlit, ZenML Dashboards.
Trainer Details - MLOPS Training in Hyderabad
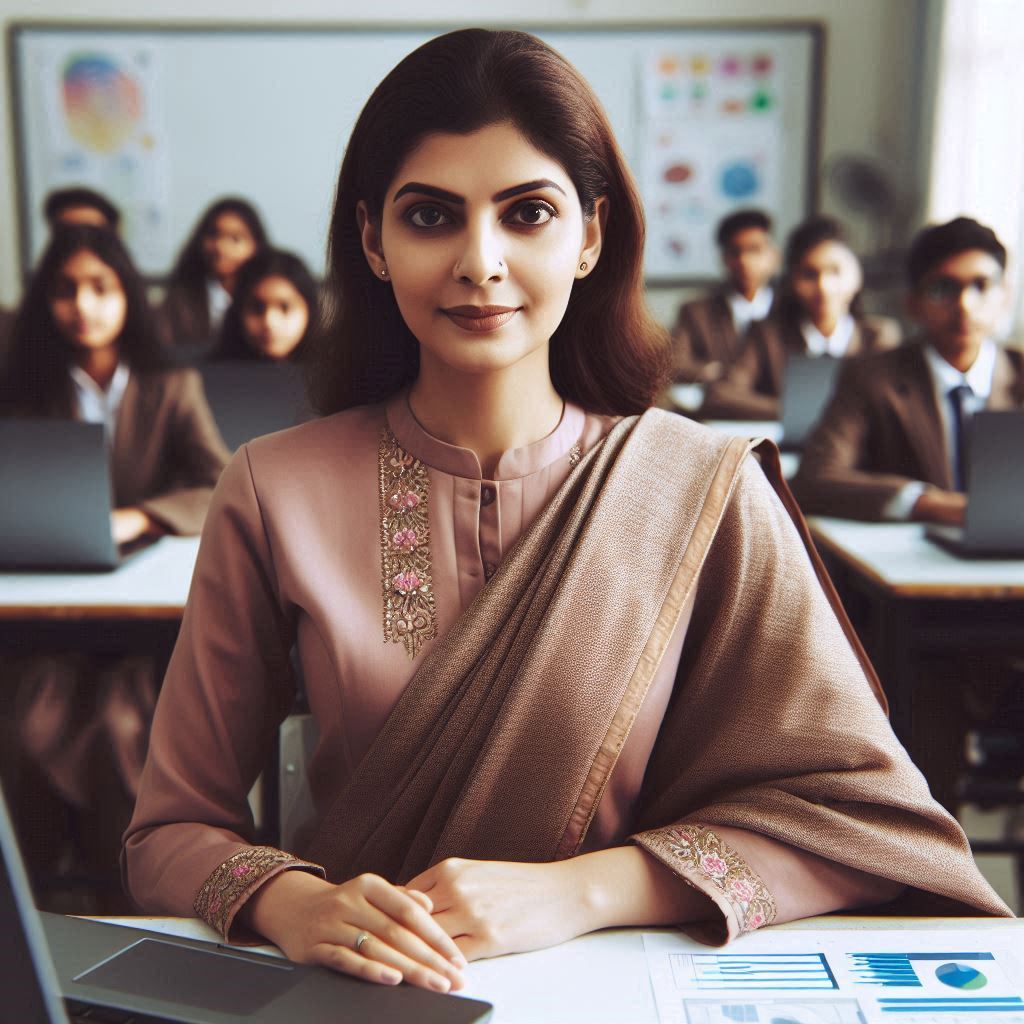
Ms. Madhumathi
Principal Data Scientist & Generative AI Strategist
10+ Years of Experience
About The Trainer
As an industry expert in Generative AI and an accomplished lead trainer, she is dedicated to advancing students’ careers by integrating real-time applications into Data Science and Generative AI education.
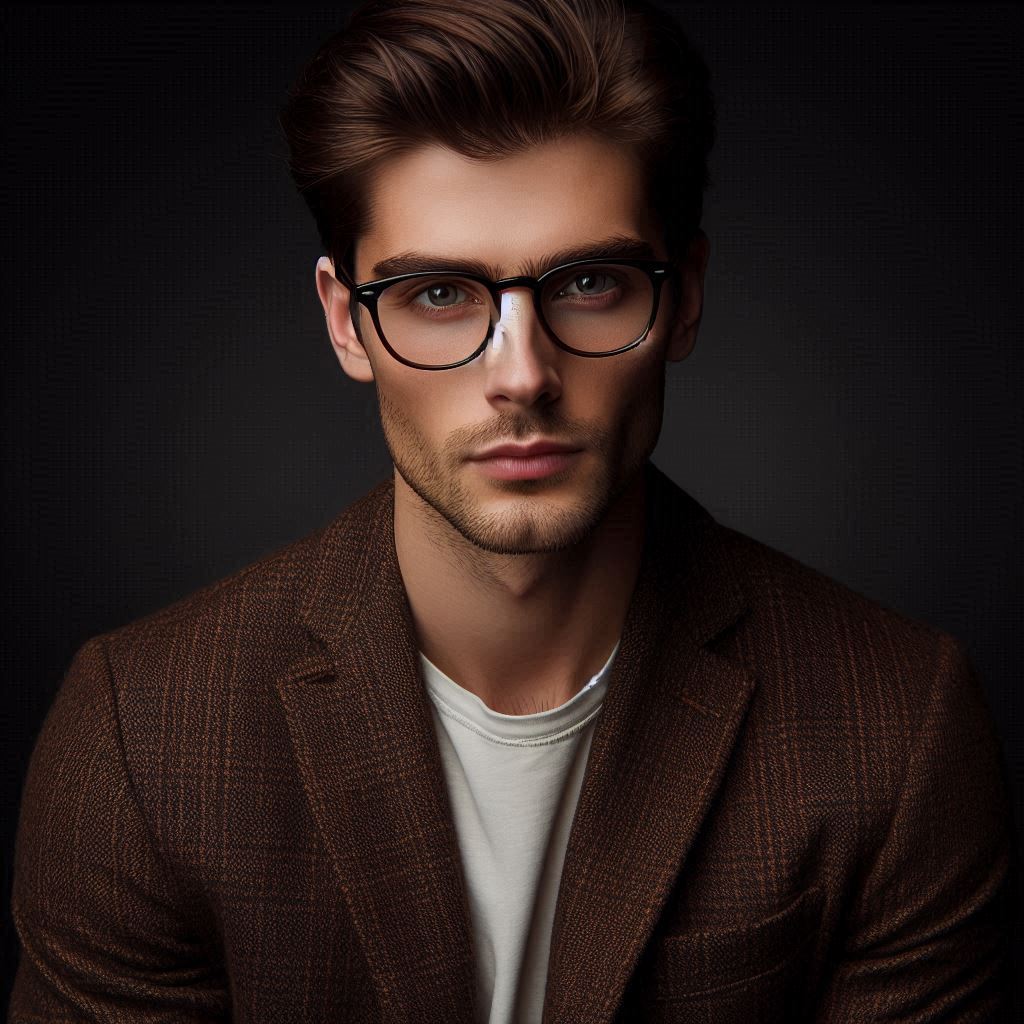
Mr. Prasad
Generative AI Authority & Principal Data Scientist
20+ years of Experience
About The Trainer
Our trainer is an Expert in AI specialist and Lead Data Scientist with deep expertise in Machine Learning, Deep Learning, NLP, Python/R, along with Statistical, Biological, and Panel Analysis.
The trainer has extensive experience in the healthcare and medical fields, having managed projects across the US, UK, Australia, and Canada. He possesses strong expertise in image processing for various diseases, utilizing Generative AI techniques.
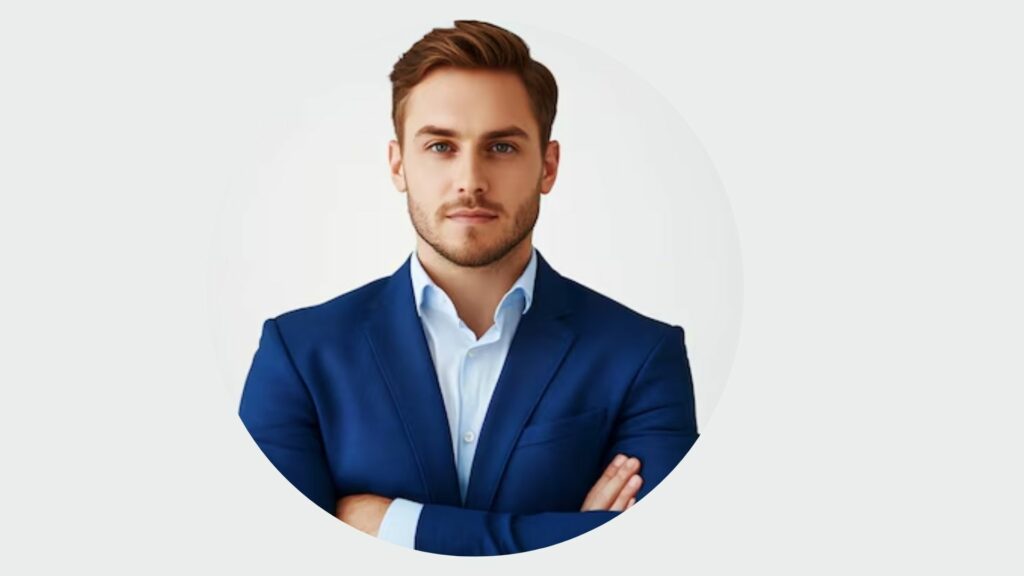
Mr. Rehan
Generative AI Projects ,Student Doubt Solver
15+ years of Experience
About the Trainer
Our trainer is an Expert in Generative Ai project’s and student doubt solver .He Helps students build real-time Generative AI Projects that are useful and practical. He is always ready to solve students’ questions with easy explanations and full support.
Uses simple English, real examples, and live demos to make AI topics easy to understand. Many students who trained under Mr. Rehan have improved their skills and gained confidence. Mr. Rehan regularly learns new AI tools and shares the latest updates with students. Very friendly, kind, and patient while teaching. Students feel free to ask any doubts.
Why Choose us
Expert Instructors
Our Training is led by industry professionals with strong hands-on experience in MLOps and machine learning. They share real-world knowledge, offer practical examples, and provide continuous guidance to help learners understand concepts clearly and apply them effectively.
Hands-On Experience
At Generative AI Masters, you’ll learn by doing. You’ll work on real projects and examples that help you understand how MLOps works in real life. This practical training builds your skills and helps you feel more confident using MLOps tools and methods.
Complete Curriculum
Generative AI Masters provides a clear and organized MLOps course. It covers everything from basic to advanced topics. You’ll also get hands-on practice with popular tools like Docker, Kubernetes, and cloud platforms, so you can fully understand how MLOps works in real-world situations.
Industry-Relevant Training
The course is Designed based on what companies are currently looking for. You’ll learn the skills and tools that are in demand, which can help you find better job opportunities in the MLOps field.
Personalized Support
Generative AI Masters gives each student personal help and guidance. You’ll get support with your projects, career tips, and help if you face any difficulties during the course.
Strong Placement Support
Generative AI Masters provides solid help with job placement. They offer career advice, resume tips, and support with interviews. Their connections with companies also help students find good job opportunities in the MLOps field.
Flexible Learning Options
The institute offers both online and offline classes, so you can choose what works best for your schedule. This flexibility makes it easier for working professionals to learn without interrupting their jobs.
Positive Reviews and Success Stories
Many students who completed the training at Generative AI Masters have moved forward in their MLOps careers. The good feedback and success stories show that the program really works and helps people succeed.
Modes - Generative AI Course in Hyderabad
Classroom Training
- Interactive Face-to-Face Teaching
- Industry Expert Trainers
- Instant Feedback
- Collaborative Tasks
- Hands-on Industry Projects
- Group Discussions
- Covers Advanced Topics
Online Training
- Virtual Learning Sessions
- Daily Session Recordings
- Instructor Support
- Interactive Webinars
- Digital Learning Modules
- Online Practical Labs
- Flexible Learning Schedules
Corporate Training
- Customized Training Programs
- Daily Recordings
- Interactive Team Development
- Expert Instruction
- Industry-Relevant Content
- Performance Monitoring
- On-Site Workshops
What is MLops ?
- MLOps stands for Machine Learning Operations. It combines the processes of machine learning and DevOps to make it easier to build, test, deploy, and manage ML models in production environments.
- It focuses on automating and improving the process of deploying machine learning models, making it quicker, more reliable, and easier to manage in production environments.
- MLOps manages the full machine learning workflow — starting from preparing the data, training the model, deploying it, and continuing through to tracking and monitoring its performance after deployment.
- It helps data scientists and IT operations teams work together more effectively by creating smoother communication and shared workflows throughout the machine learning process.
- Continuous Integration and Continuous Delivery (CI/CD) are important parts of MLOps. They help automate testing, building, and deploying machine learning models, making the entire process faster, more reliable, and easier to update as needed.
- MLOps makes it easier to update and scale machine learning models quickly and reliably, ensuring they stay accurate and can handle growing amounts of data or users without issues.
- It involves monitoring models once they are in production to detect problems like data drift and fix them promptly.
- The main goal of MLOps is to make machine learning models more dependable and simpler to maintain when used in real-time applications.
Why is MLops Used?
- MLOps is used to make it easier and faster to put machine learning models into real use.
- It helps make sure machine learning models are updated in a steady and trustworthy way.
- MLOps watches models that are being used, so problems can be found and fixed quickly.
- It helps data scientists and IT teams work together better.
- MLOps makes it easier to keep adding and improving machine learning models.
- It helps machine learning models work well even when used by many people at the same time.
- MLOps makes it simple to manage changes in data and update models.
- It helps machine learning systems work more smoothly and without problems.
About MLops
MLOps, short for Machine Learning Operations, is a practice that combines machine learning (ML) with DevOps to streamline and automate the process of deploying, monitoring, and managing machine learning models in production.
It bridges the gap between data scientists and IT operations, ensuring that machine learning models can be efficiently and reliably integrated into real-time applications.
MLOps includes the entire machine learning lifecycle, including data preparation, model training, deployment, monitoring, and retraining, allowing organizations to continuously deliver and improve ML-driven solutions.
The primary goal of MLOps is to enhance the collaboration between data science teams and operations teams, enabling faster experimentation, more reliable deployments, and better scalability.
By applying principles from DevOps, such as continuous integration and continuous delivery (CI/CD), MLOps ensures that models can be rapidly tested and deployed, with automated workflows reducing the risk of errors.
Additionally, MLOps emphasizes the importance of monitoring models in production to detect issues like data drift or performance degradation, allowing for timely interventions and model updates.
This approach not only accelerates the deployment of machine learning models but also ensures their long-term reliability and effectiveness in production environments.
Generative AI Masters in Hyderabad offers MLOps training designed to equip learners with the skills needed to operationalize machine learning models effectively.
The course covers essential tools and practices like Kubernetes, Docker, Jenkins, and cloud platforms such as AWS and Azure, ensuring participants gain hands-on experience in automating and managing ML pipelines.
With a curriculum that blends theory with practical application through real-world projects, Generative AI Masters prepares both beginners and professionals to excel in the fast-growing field of MLOps, guided by expert instructors who provide in-depth knowledge and industry insights.
Course Outline
01
The course starts with an introduction to MLOps concepts and its importance in machine learning.
02
03
04
Students get practical experience using CI/CD methods to build and update machine learning models.
05
06
07
08
Tools Covered
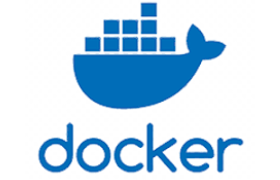
Docker
Used to wrap machine learning applications so they run the same way in development, testing, and production.
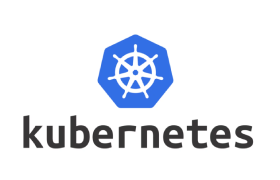
Kubernetes
Used to manage and control containerized applications, making it easier to deploy and scale machine learning models.
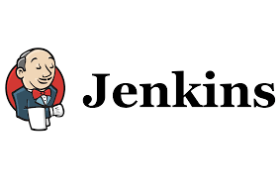
Jenkins
Used to automate the building, testing, and delivery process for machine learning models.
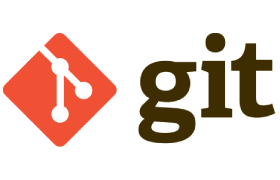
Git
Used to keep track of changes in code and model settings.
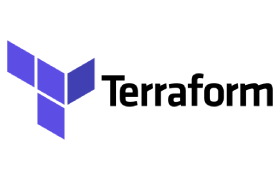
Terraform
Used to automatically create and manage cloud resources with code.
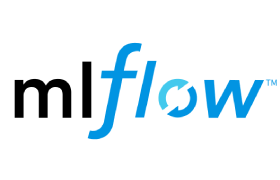
MLflow
Used to track experiments, manage different model versions, and help deploy models.
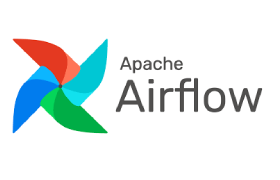
Apache Airflow
Used to schedule and keep an eye on tasks and pipelines in machine learning projects.
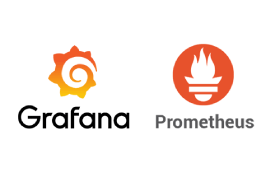
Prometheus and Grafana
Used to monitor and show graphs of model performance and system health.
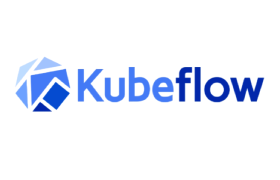
Kubeflow
Used to deploy and manage machine learning workflows on Kubernetes.
Skills developed post MLops training
- Use Tools Like Docker & Kubernetes You’ll know how to use tools that help package and run machine learning models easily across different systems.
- Automate ML ProcessesYou’ll be able to set up systems that automatically handle tasks like training, testing, and updating models — saving time and reducing errors.
- Understand CI/CD for ML You’ll learn how to keep your machine learning projects running smoothly with processes that automatically test and update your work.
- Work with Cloud PlatformsYou’ll get hands-on experience deploying machine learning models on cloud services like AWS and Azure, so your models can scale as needed.
- Monitor Models in Production You’ll know how to keep an eye on your models after they’re deployed to make sure they continue to perform well over time.
- Handle Data and Features Properly You’ll learn how to manage different versions of your data and engineer useful features, helping your models stay accurate and effective.
- Fix Model IssuesWhen a model starts performing poorly or behaving differently, you’ll have the skills to figure out what’s wrong and fix it.
- Work Well with TeamsYou’ll understand how to collaborate better with both data science and IT teams — helping projects move faster and smoother.
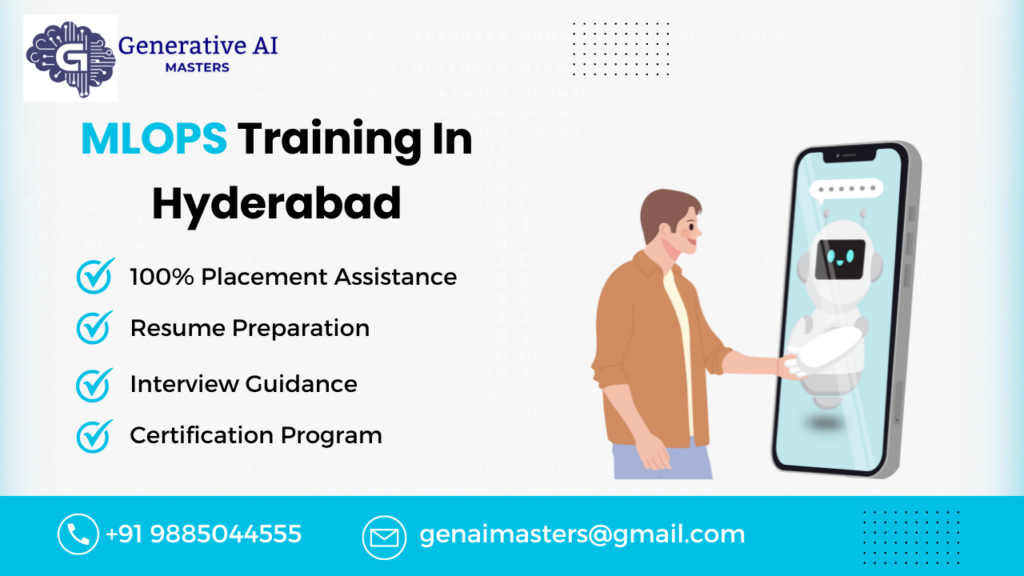
Career Opportunities in MLOps
MLOps is one of the fastest-growing areas in tech, offering a wide range of career paths for professionals who can manage and optimize machine learning in real-world environments. With the increasing adoption of AI across industries, skilled MLOps professionals are in high demand.
Here are some key job opportunities in MLops
- MLOps Engineer Responsible for deploying, managing, and scaling machine learning models in production. Acts as a bridge between data science and IT operations to ensure seamless integration.
- Machine Learning Operations Specialist Focuses on automating ML workflows, maintaining model performance, and applying best practices to keep systems running efficiently.
- Data Engineer Builds and manages the data pipelines that feed into machine learning models, ensuring high-quality, reliable data is always available.
- DevOps EngineerIntegrates ML models into existing DevOps processes, working on CI/CD pipelines, infrastructure, and deployment automation.
- ML Infrastructure Engineer Designs and manages the technical infrastructure — including cloud and on-premise resources — required to support ML workloads.
- AI Operations ManagerOversees the full machine learning operations lifecycle, from deployment to monitoring, ensuring models stay accurate and up to date
- Machine Learning Architect Plans and designs the overall architecture for scalable and reliable ML model deployment, focusing on performance and integration.
- Data Scientist with MLOps Skills Combines data science expertise with MLOps know-how to not only build models but also make them production-ready and maintainable.
- Cloud Engineer Manages cloud infrastructure for ML applications, enabling scalable and secure deployment of models on platforms like AWS, Azure, or GCP.
- Model Monitoring Specialist Specializes in tracking how ML models perform in real time, detecting issues such as data drift or performance decline, and triggering corrective actions.
Key Points of MLops
- The MLOps training at Generative AI Masters focuses on integrating machine learning with DevOps practices.
- It provides hands-on experience with tools like Kubernetes, Docker, and Jenkins.
- The course covers end-to-end machine learning pipeline automation.
- Students learn about continuous integration and continuous deployment (CI/CD) for machine learning models.
- The training includes real-world projects to apply MLOps concepts in practical scenarios.
- The curriculum is designed to help participants master model monitoring and management.
- It offers insights into data versioning and feature engineering for robust ML models.
- Generative AI Masters' MLOps course includes training on cloud platforms like AWS and Azure.
- The program is suitable for both beginners and professionals looking to enhance their skills in MLOps.
- Expert instructors guide students through the complexities of operationalizing machine learning models.
Companies That Hire
Placement Support at Generative AI Masters
At Generative AI Masters, we believe that learning is just the beginning — our goal is to help you build a successful career in the world of MLOps and AI. That’s why we offer a complete placement support program as part of our training.
Our placement program is designed to guide and support you through every step of your job search, making sure you’re fully prepared to land the right role with confidence.
Here’s What You’ll Get With Our Placement Program
Career Counseling
We offer personalized career guidance to help you figure out the best path based on your skills, interests, and long-term goals. Whether you’re starting fresh or switching careers, our mentors will help you choose the right direction in MLOps or related roles.
Resume Building
A strong resume is your first step into any job. Our team will work with you to create a professional resume that highlights your training, hands-on projects, technical skills, and certifications — everything recruiters are looking for.
Interview Preparation
Interviews can be stressful, but we make sure you’re ready. You’ll go through mock interviews, get feedback on your answers, and learn how to tackle both technical questions and HR rounds with confidence. We’ll also share tips and common questions asked by top companies.
Job Search Support
We don’t stop at training — we actively help you find opportunities. You’ll get access to job listings, company referrals, and placement leads through our network of partner companies in the MLOps, AI, and machine learning industry. We’ll also help you apply for jobs that match your profile.
MLOPS Training in Hyderabad
4000+ Jobs Opening For MLOPS
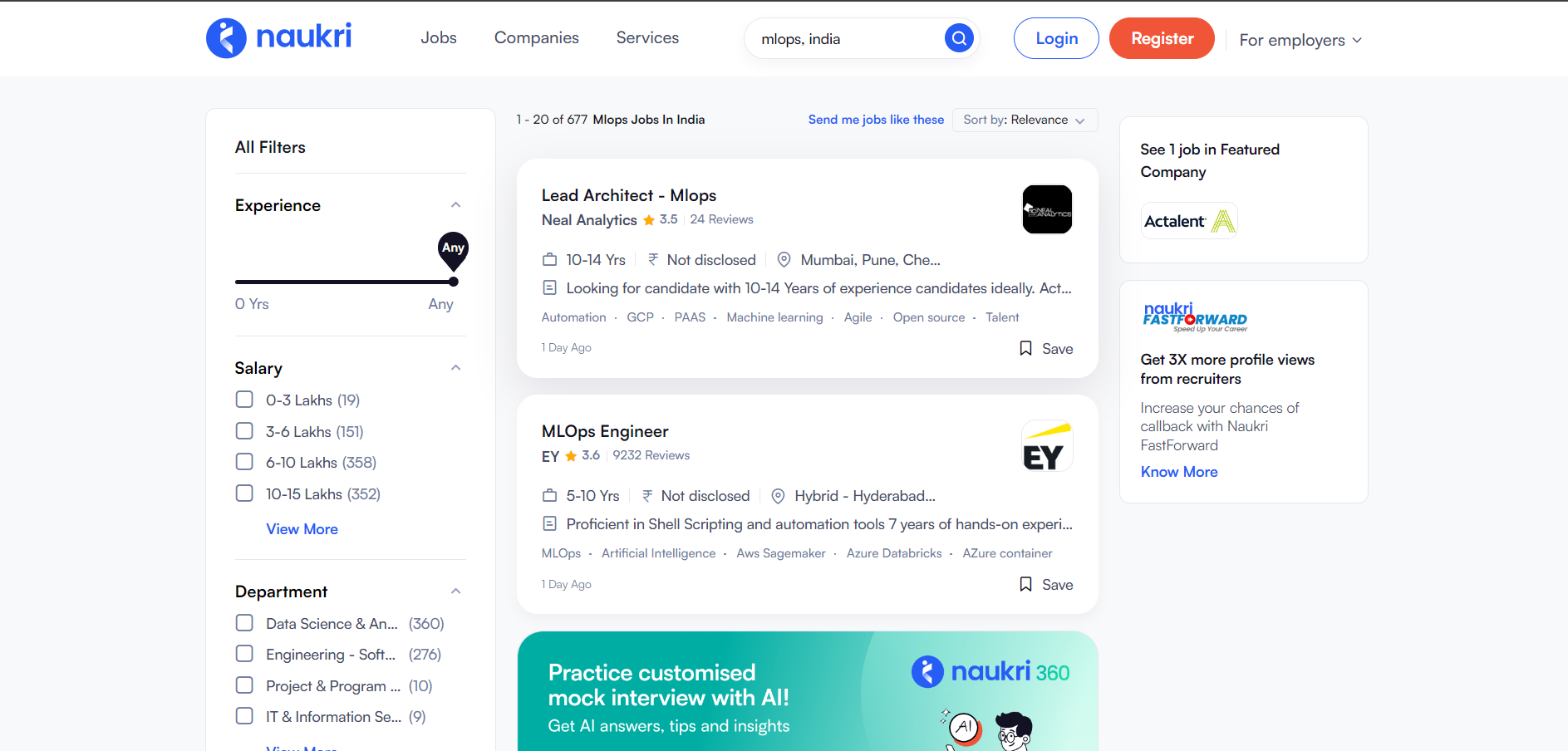
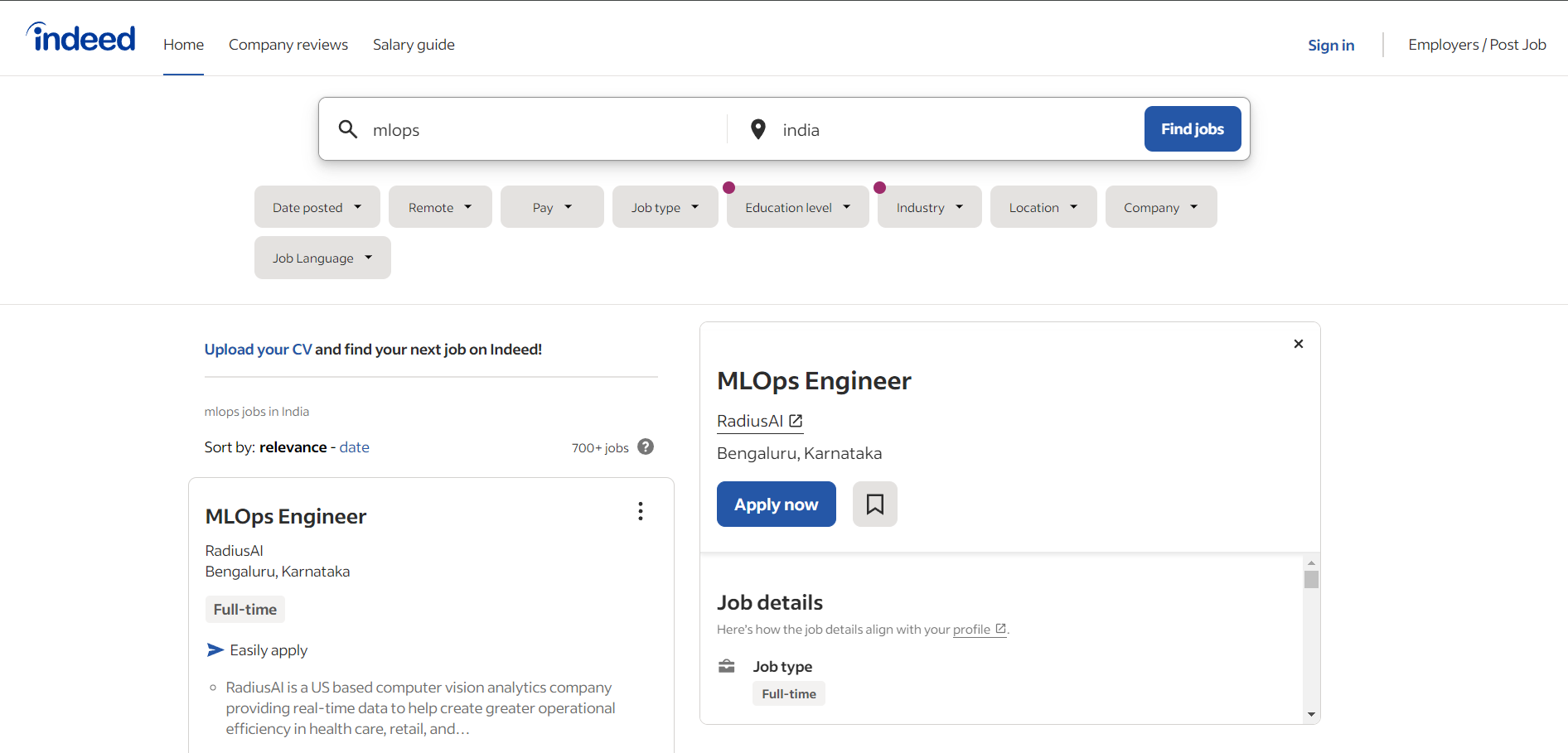
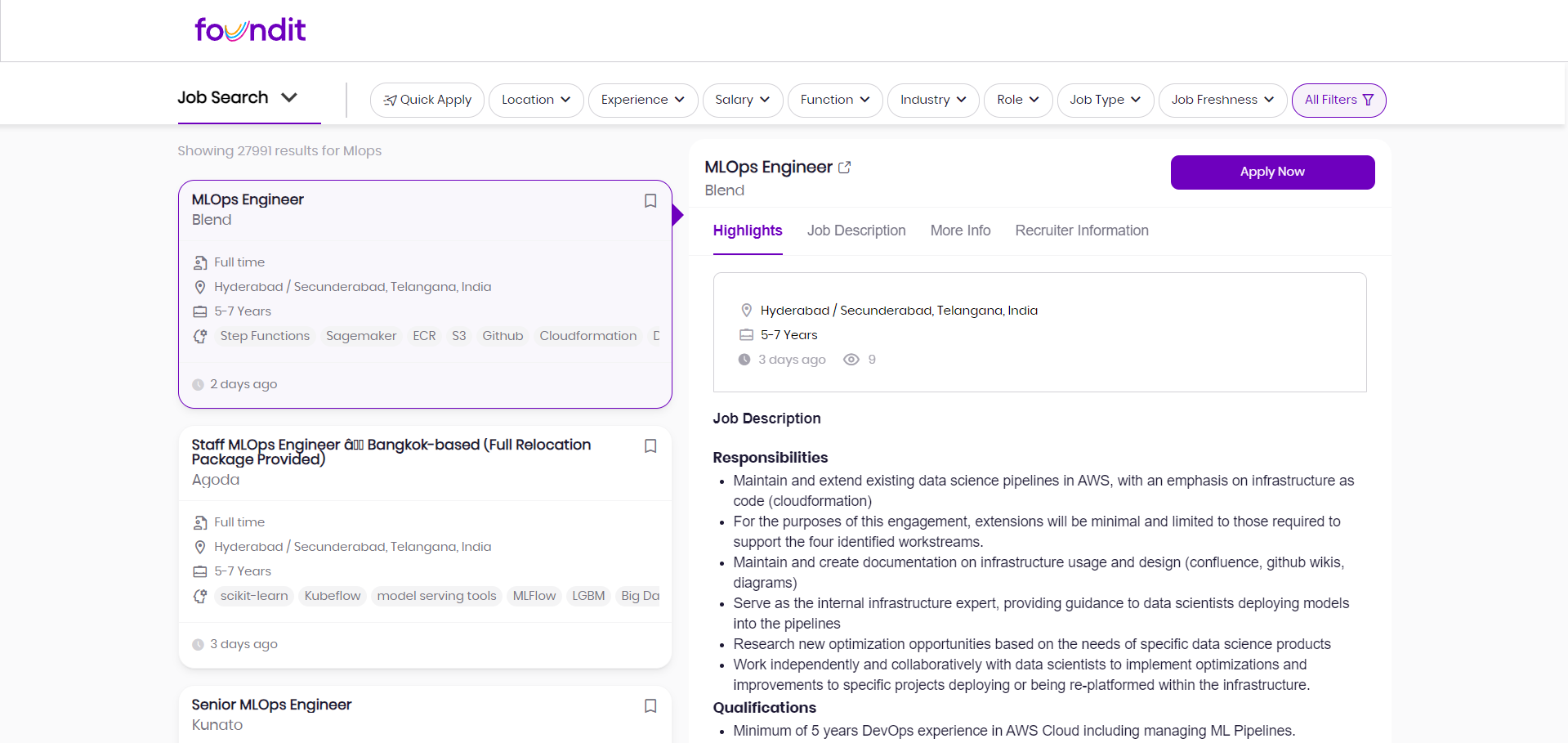
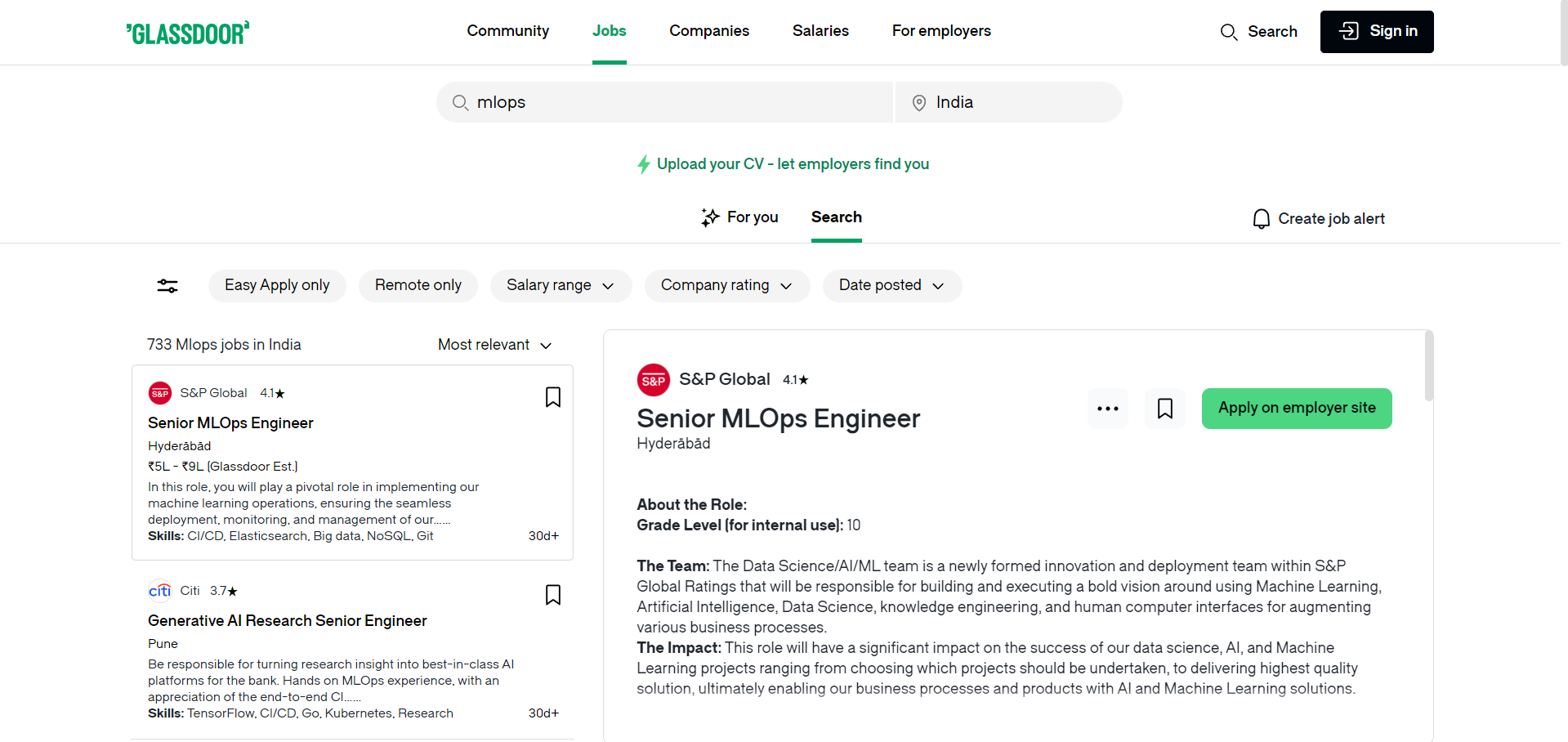
Prerequisites
You don’t need to be an expert to join — just a few basics will help you get the most out of the training
- Basic Machine Learning KnowledgeKnow a little about how machine learning works — like what models are and how they learn from data.
- Python ProgrammingYou should be comfortable writing simple code in Python, since we’ll use it throughout the course.
- Using Git (Helpful, Not Required)If you’ve used Git to manage code before, that’s great! If not, we’ll help you get started.
- Cloud or Docker Experience (Nice to Have)If you’ve worked with cloud platforms like AWS or used tools like Docker, it’s a plus — but not necessary.
Don’t worry if you’re missing something — we provide support and extra resources to help you catch up!
Approximate Pay Scale in MLOPS Engineer
Entry-Level MLOps Engineer
- Experience: 0–2 years
- Salary Range: 6-10 lakhs per Annum
- Responsibilities
- Help with setting up and running machine learning models
- Assist in automating simple ML tasks and workflows
- Learn and use tools like Docker, Git, and CI/CD systems
- Work with data scientists and DevOps teams on daily tasks
- Keep project files organized and well-documented
- Help with setting up and running machine learning models
Mid-Level MLOps Engineer
- Experience: 2–5 years
- Salary Range: 12-18 lakhs per Annum
- Responsibilities
- Build and manage pipelines for training and deploying models
- Improve the speed and reliability of model updates
- Use cloud platforms (like AWS, Azure, or GCP) to manage systems
- Set up tools to monitor models and spot performance issues
- Guide junior team members and lead small projects
- Build and manage pipelines for training and deploying models
Senior MLOps Engineer
- Experience: 5+ years
- Salary Range: 20-35 lakhs per Annum
- Responsibilities
- Lead the design of full MLOps systems from start to finish
- Create rules and best practices for tools and workflows
- Oversee how models are tracked, updated, and rolled back if needed
- Make sure systems are safe, stable, and follow regulations
- Mentor others and help shape the technical direction of the team
- Lead the design of full MLOps systems from start to finish
Lead or MLOps Architect
- Experience: 7+ years
- Salary Range: 38-43 lakhs per Annum
- Responsibilities
- Plan and lead the company’s overall MLOps strategy
- Design large and scalable machine learning systems
- Manage teams across data science, Engineering, and DevOps
- Choose and set up the best tools and technologies for the job
- Make sure MLOps practices support the company’s business goals
- Plan and lead the company’s overall MLOps strategy
Market trend
MLOps (Machine Learning Operations) is quickly becoming one of the most in-demand fields in the AI and data world. As more companies adopt machine learning, there’s a growing need for skilled professionals who can help deploy, manage, and scale ML models effectively.
- Rapid Growth and DemandWith machine learning being used across industries — from healthcare to finance — companies are actively looking for experts in MLOps to manage and maintain their AI systems.
- Automation Is KeyBusinesses want to reduce manual work and errors in deploying models. That’s why automating ML workflows and model updates has become a top priority — and MLOps makes it possible.
- Cloud + MLOps = Scalability Most companies are moving their ML infrastructure to the cloud. Integrating MLOps with cloud platforms like AWS, Azure, and GCP helps make these systems more scalable, efficient, and cost-effective.
- New Tools and Frameworks Are EmergingAs the field grows, so does the technology. New MLOps tools and platforms are being developed to solve challenges like model monitoring, version control, and real-time performance tracking.
- Focus on Model Monitoring and ManagementDeploying a model is just the beginning. Organizations now realize the importance of continuously monitoring model performance and handling issues like data drift or model degradation over time.
-
Rising Job Opportunities
Roles like MLOps Engineer, Data Engineer, ML Infrastructure Specialist, and AI Operations Manager are becoming more common — and are some of the most exciting and high-paying roles in tech today. - Bridging the Gap Between TeamsMLOps professionals play a key role in connecting data science teams (who build models) with IT/DevOps teams (who deploy and manage them). This collaboration is crucial for AI success in real-world projects.
Generative AI Masters achievements
Our Great Achievements
Generative AI Learners Testimonials
Certification
Certifications in MLOps are valuable for professionals looking to validate their skills and knowledge in managing machine learning operations. These certifications demonstrate proficiency in deploying, monitoring, and maintaining machine learning models, and they can significantly enhance career prospects.
Earning a certification often involves passing an exam that tests understanding of key concepts, tools, and best practices in MLOps, such as continuous integration, continuous deployment (CI/CD), and model monitoring.
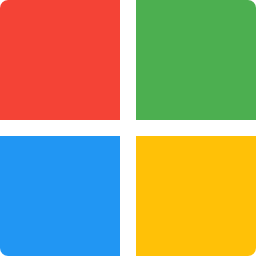
Microsoft Certified: Azure Data Scientist Associate
This certification focuses on managing machine learning models and data pipelines using Microsoft Azure. It covers key aspects of deploying, managing, and optimizing ML solutions in the Azure environment.
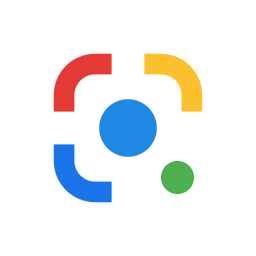
Google Professional Machine Learning Engineer
This certification demonstrates expertise in designing, building, and deploying ML models using Google Cloud Platform. It emphasizes practical skills in managing machine learning solutions and ensuring their scalability and performance.
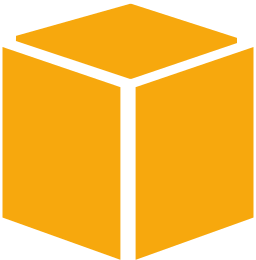
AWS Certified Machine Learning – Specialty
This certification showcases proficiency in deploying and managing machine learning models on Amazon Web Services (AWS). It covers topics such as model optimization, deployment, and maintenance in the AWS ecosystem.
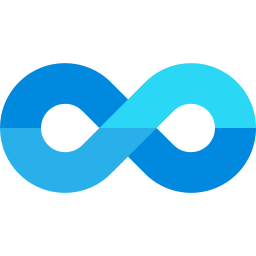
Certified MLOps Professional (CMOP)
The CMOP certification is specifically designed for MLOps practitioners. It focuses on best practices and tools for operationalizing machine learning models, including model deployment, monitoring, and lifecycle management.
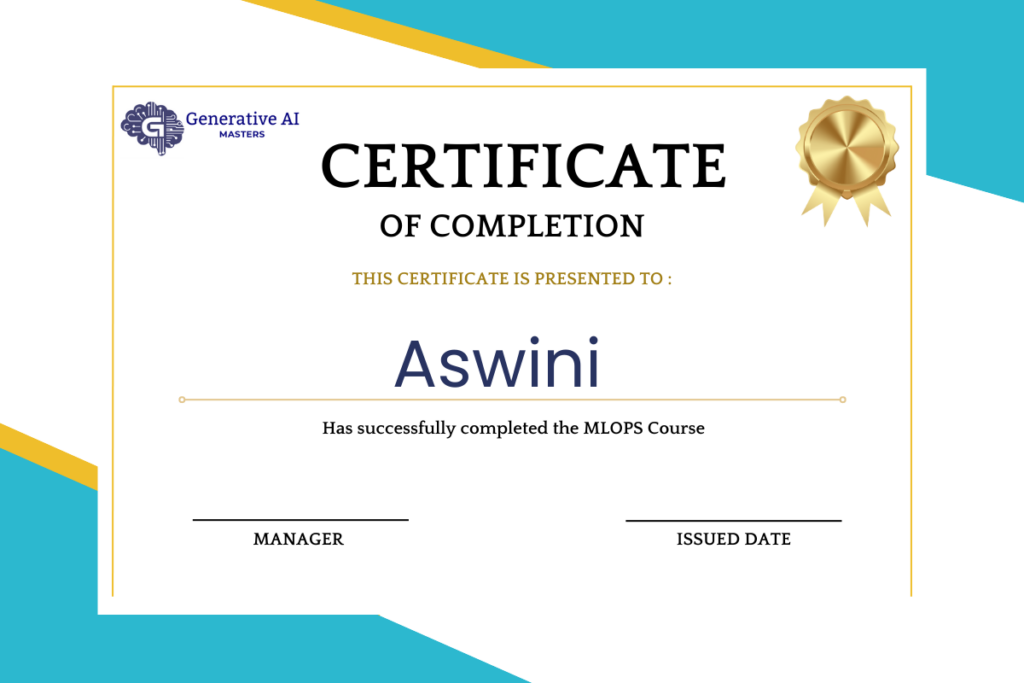
Faqs
MLOps, or Machine Learning Operations, is a set of practices that combines machine learning with DevOps to streamline and automate the deployment, management, and monitoring of machine learning models in production environments.
MLOps training helps professionals gain skills in automating ML pipelines, managing model deployment, monitoring performance, and integrating ML models with existing IT infrastructure, leading to improved efficiency and scalability.
Basic understanding of machine learning concepts, familiarity with Python programming, and knowledge of version control systems like Git are recommended prerequisites. Experience with cloud platforms or containerization tools is helpful but not required.
The duration of MLOps training programs can vary, but typically they range from a 3 – 4 months, depending on the depth of the course and the learning format.
MLOps training often includes tools like Docker, Kubernetes, Jenkins, Git, MLflow, Apache Airflow, and cloud platforms such as AWS, Azure, and Google Cloud.
Yes, MLOps training can be suitable for beginners who have a basic understanding of machine learning and programming. Many programs are designed to cater to varying levels of experience.
Career opportunities include roles such as MLOps Engineer, Machine Learning Operations Specialist, Data Engineer, DevOps Engineer, ML Infrastructure Engineer, and AI Operations Manager.
The salary of an MLOps Engineer varies based on their skillset. Recent reports suggest that, on average, MLOps Engineer earn ₹13,00,000 per annum.
Yes, Generative AI Masters provides placement assistance, including career counseling, resume building, interview preparation, and access to job opportunities through its industry network for more Details contact Gen Ai masters .
Yes, some MLOps training programs may prepare you for certifications such as the Microsoft Certified: Azure Data Scientist Associate, Google Professional Machine Learning Engineer, AWS Certified Machine Learning – Specialty, and Certified MLOps Professional (CMOP).
Yes, many MLOps training programs are offered online, providing flexibility to learn from anywhere. These programs often include virtual classrooms, recorded sessions, and online resources.
MLOps is used to automate, monitor, and manage machine learning workflows, ensuring smooth deployment, scalability, and maintenance of ML models in production environments.
- DevOps focuses on software development and operations.
- MLOps adds ML-specific tasks like data processing, model training, and versioning on top of DevOps to support ML lifecycles.
MLOps is a great career choice because many companies are starting to use AI more. There’s a strong need for people with MLOps skills. It also offers good salaries, chances to grow in your career, and opportunities to work with the latest technologies.
- CI (Continuous Integration) automates testing and versioning of ML code/models.
- CD (Continuous Delivery/Deployment) automates the process of deploying models to production.
Yes. Kubernetes is commonly used to deploy, scale, and manage ML workloads, especially in containerized environments.
MLOps certifications validate your skills in ML model deployment, automation, and pipeline management. Examples include
- Google Cloud MLOps Engineer
- AWS Machine Learning Certification
- Coursera/edX/Databricks MLOps courses
MLOps will become a core part of AI infrastructure, with more automation, better monitoring, and tighter integration with data pipelines and edge computing.
- Automation of pipelines
- Version control for data, models, and code
- Reproducibility
- Continuous training & deployment
- Monitoring & governance
- Collaboration across teams
Yes, but not as much as data science. Knowledge of Python, scripting, and tools like Docker, Kubernetes, and CI/CD pipelines is essential.