Generative AI in Healthcare
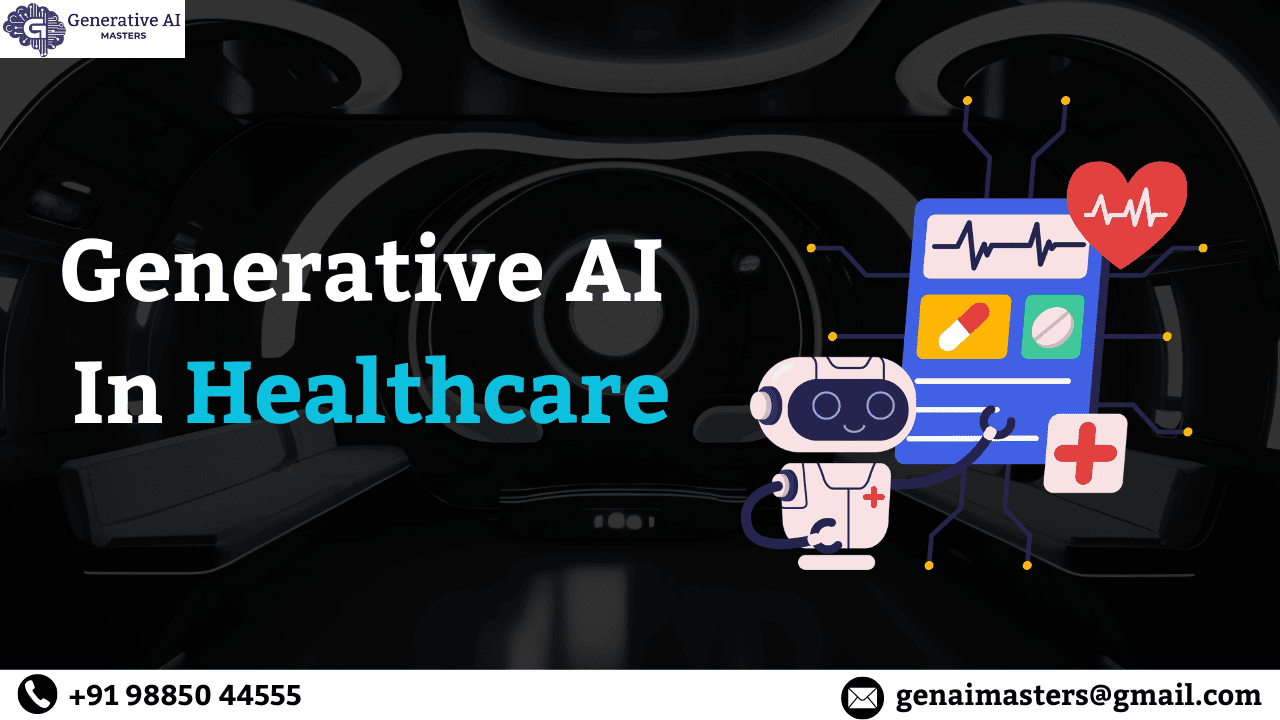
Generative AI in Healthcare
Introduction
Understanding Generative AI
What is Generative AI?
Generative AI refers to a type of artificial intelligence that can produce new content by learning from existing datasets. Unlike traditional machine learning models that focus primarily on classification and prediction tasks, generative AI creates novel outputs such as text, images, or even music based on learned patterns from training data. This ability makes it particularly useful in fields like healthcare, where it can generate synthetic patient data or assist in developing new treatment protocols.
At its core, generative AI relies on advanced algorithms like Generative Adversarial Networks (GANs) and Variational Autoencoders (VAEs). GANs consist of two neural networks—a generator and a discriminator—that work against each other to create realistic outputs. The generator creates new data instances while the discriminator evaluates them against real data. Through this adversarial process, the generator improves its ability to produce authentic-looking outputs over time.
Generative AI’s applications are vast; it can be used to create synthetic medical images for training purposes or develop personalized treatment plans based on an individual’s unique health data. The technology has also shown promise in predicting disease outbreaks by analyzing social media trends and other public data sources.
As the healthcare industry increasingly embraces digital transformation, understanding the fundamentals of generative AI becomes essential for practitioners and organizations alike. By leveraging this technology effectively, healthcare providers can improve patient outcomes while also streamlining operational processes.
Key Features of Generative AI
Generative AI possesses several key features that make it particularly valuable in healthcare settings:
- Content Creation: The ability to generate text or images based on user prompts allows for personalized communication with patients and tailored educational materials.
- Predictive Modeling: Generative AI can create models that predict health outcomes based on historical data. This capability is crucial for early diagnosis and preventive care.
- Data Synthesis: By producing synthetic data that mimics real patient information without compromising privacy, generative AI can be used for training other machine learning models.
These features enable healthcare providers to enhance their services significantly while addressing common challenges such as high operational costs and inefficiencies
Applications of Generative AI in Healthcare
Clinical Decision Support
Generative AI is making significant strides in clinical decision support systems (CDSS). These systems are designed to assist healthcare providers in making informed decisions by analyzing complex datasets and presenting actionable insights quickly.
For instance, when faced with complicated cases involving multiple health issues, clinicians may struggle to recall all relevant studies or treatment guidelines. Generative AI can analyze patient history alongside current research to suggest evidence-based treatment options tailored to individual needs. This capability not only improves diagnostic accuracy but also enhances patient safety by reducing the likelihood of human error.
Moreover, generative AI can facilitate real-time monitoring of patient vitals through wearable devices. By continuously analyzing incoming data streams, the system can alert healthcare providers about potential complications before they escalate into serious issues. This proactive approach allows for timely interventions that can significantly improve patient outcomes.
Additionally, generative AI can help identify trends within large populations by analyzing electronic health records (EHRs). For example, it can uncover correlations between lifestyle factors and disease prevalence, enabling public health officials to implement targeted interventions aimed at reducing health disparities.
As more healthcare organizations adopt generative AI-driven CDSS tools, we expect to see a shift toward more collaborative decision-making processes where clinicians work alongside intelligent systems rather than relying solely on their expertise.
Improved Drug Discovery
Researchers can input specific parameters related to desired drug characteristics, such as efficacy against a particular disease or reduced side effects, and then allow the algorithm to generate potential compounds that meet these criteria. Recent progress in this field has led to significant breakthroughs. As of December 2023, approximately 70 drugs developed with the assistance of generative AI were undergoing clinical trials in various therapeutic areas, including oncology, neurology, and infectious diseases.
Enhancing Patient Engagement
Patient engagement is crucial for improving health outcomes; however, many patients struggle with navigating complex healthcare systems or understanding their treatment options fully. Generative AI enhances patient engagement through innovative communication tools such as chatbots and virtual assistants that provide personalized support around-the-clock.
These chatbots can answer common questions about medications or treatment plans while collecting vital health information from patients during interactions—helping clinicians make informed decisions based on up-to-date insights into each patient’s condition. Furthermore, they enable seamless appointment scheduling or prescription refills without requiring direct human intervention.
Personalized communication extends beyond chatbots; generative AI can also create tailored educational materials based on individual patient profiles—ensuring that patients receive relevant information about their conditions at appropriate reading levels or formats (e.g., videos versus text).
As telehealth continues gaining traction post-pandemic—providing remote consultations between patients and providers—generating personalized follow-up messages or reminders becomes increasingly important for maintaining engagement throughout care journeys.
Ultimately,enhanced patient engagement fosters greater adherence to treatment plans while empowering individuals with knowledge about their health—leading not only to improved outcomes but also increased satisfaction levels across diverse populations.
Streamlining Administrative Tasks
Administrative burdens are among the leading causes of clinician burnout within healthcare settings today—distracting providers from delivering quality care while contributing significantly toward rising operational costs across organizations nationwide. Fortunately,generativе AIs offer solutions aimed at streamlining these tedious tasks through automation capabilities designed specifically for administrative workflows.
For example,generativе AIs excel at transcribing conversations during consultations—allowing clinicians’ attention remain focused entirely upon patients rather than note-taking duties which often detract from meaningful interactions during visits。This capability not only saves time but also reduces errors associated with manual documentation processes。
Additionally,generativе AIs facilitate drafting clinical notes based upon structured templates derived directly from EHRs。By pre-filling pertinent details regarding diagnoses,treatment plans,and follow-up recommendations,these tools enable quicker completion times while ensuring consistency across documentation efforts。
Further more, managing electronic health records (EHRs) becomes more efficient when leveraging automated systems capable of organizing files according specific criteria such as date ranges or specialties。This organization enhances access speed when retrieving critical information needed during urgent situations。
By alleviating administrative burdens through automation technologies like those powered by generativе AIs,clinicians regain valuable time previously lost due tedious paperwork—ultimately allowing them devote greater attention toward providing high-quality care experiences for all patients served.
Benefits of Generative AI in Healthcare
Enhanced Efficiency
The incorporation of generative AIs into healthcare operations holds great promise for significantly boosting efficiency levels across various areas, from clinical workflows to administrative processes. One key advantage is the automation of routine tasks that traditionally consume substantial amounts of time without directly contributing to patient care delivery.
For example, by automating appointment scheduling systems using intelligent algorithms capable of predicting optimal times based on historical patterns observed across similar cases, healthcare organizations can reduce wait times for patients seeking services. This optimization not only enhances client satisfaction but also maximizes the utilization of resources available within the facilities.
Furthermore, generative AIs improve efficiency in diagnostic processes by rapidly analyzing large datasets encompassing diverse variables that influence health outcomes. By identifying trends within these datasets more quickly compared to traditional methods reliant solely on human interpretation, clinicians receive timely insights informing decisions about treatments for individuals diagnosed with various conditions.
Moreover, streamlined documentation efforts enabled by automated transcription tools significantly reduce the time spent completing paperwork. This presents a win-win scenario benefiting both providers seeking to minimize burnout levels associated with excessive workloads and ensuring accurate records are maintained throughout the continuum of care delivered to each patient.
In summary, the enhanced efficiency achieved through effective implementation strategies surrounding generative AIs represents a transformative opportunity to reshape how healthcare is delivered today, ultimately leading to improved experiences for both patients receiving services and providers delivering them.
If you want to learn more about Advantages of Generative AI
Improved Accuracy
“Precision is crucial in the field of medicine, and leveraging advanced technologies like generative AIs holds great potential for improving the accuracy of care delivery. These cutting-edge technologies can enhance diagnostic capabilities by quickly analyzing complex datasets to identify subtle patterns indicative of underlying health issues. For instance, generative AIs can recognize anomalies in medical imaging scans that may be overlooked by human eyes, leading to earlier detection of diseases such as cancer and ultimately improving treatment outcomes.
Generative AIs also play a role in refining predictive modeling efforts by analyzing historical data to forecast individual patients’ future health risks. By synthesizing diverse sources of information, including genetic profiles and lifestyle factors, these models provide comprehensive insights that help clinicians tailor interventions for optimal outcomes.
Additionally, the ability to generate synthetic datasets allows researchers to train machine learning models without compromising patient privacy, paving the way for more ethical and effective deployment of AI systems in practice environments.
In summary, effective integration of generative AIs offers a transformative opportunity to enhance the accuracy of diagnostics and improve the overall quality of care delivery. Embracing these strategies fully will lead to better care experiences for individuals across the entire population, ultimately reshaping the future of diagnostics in healthcare.”
Personalized Medicine
The concept personalized medicine has gained traction over recent years as advancements technologies enable more tailored approaches treating individuals based upon unique characteristics rather than one-size-fits-all methodologies employed historically across various disciplines involved managing health conditions encountered regularly among diverse populations served collectively throughout entire spectrum continuum care journey experienced every person engaged within system overall!
Generativе AIs play pivotal role facilitating this shift toward personalization through ability analyze vast amounts diverse datasets encompassing genetic profiles lifestyle factors environmental influences impacting overall well-being individuals diagnosed specific conditions encountered regularly among populations managed collectively throughout entire spectrum continuum care journey experienced every person engaged service delivery efforts undertaken collaboratively together moving forward ahead!
For example,by synthesizing information derived genetic testing results alongside historical treatment responses observed previously among similar cases encountered clinicians gain deeper understanding how best approach managing each unique case presented before them effectively ensuring optimal outcomes achieved throughout continuum care journey experienced every person served collectively across entire spectrum continuum managed overall!
Challenges and Risks Associated with Generative AI
Data Privacy Concerns
As healthcare organizations increasingly adopt generativе AIs into their workflows,data privacy concerns emerge as one critical challenge needing addressed effectively ensure compliance regulations governing protection sensitive information collected during course providing services rendered individuals engaged throughout entire spectrum continuum care journey experienced every person served collectively across entire spectrum population managed overall system-wide approach embraced fully moving forward into future ahead!
The use personal identifiable information (PII) raises ethical questions surrounding consent obtained prior utilizing such datasets training algorithms designed inform decision-making processes subsequently thereafter。 Ensuring transparency regarding how collected information utilized remains paramount fostering trust between providers patients alike while minimizing risks associated breaches occurring inadvertently exposing confidential details shared willingly during interactions held between parties involved directly engaged service delivery efforts undertaken collaboratively together moving forward ahead!
To mitigate these risks effectively requires robust governance frameworks established guiding principles adopted ensure compliance regulations governing protection sensitive information collected during course providing services rendered individuals engaged throughout entire spectrum continuum care journey experienced every person served collectively across entire spectrum population managed overall system-wide approach embraced fully moving forward into future ahead!
Bias in Algorithms
Bias in algorithms used in machine learning frameworks is a critical challenge that must be addressed to ensure fair access to quality services for all individuals. If the datasets used to train these models do not accurately represent diverse demographics, they can fail to reflect the realities faced by the communities they serve. For example, if a training dataset primarily consists of male participants aged 30-50, it may overlook the needs of women, children, and older adults. This lack of representation can lead to marginalized groups facing unequal opportunities in accessing treatment.
To mitigate these biases, intentional efforts must be made to prioritize diversity and inclusion during the design and implementation of solutions aimed at addressing the needs of specific populations. This requires a collaborative approach that involves various stakeholders in the healthcare system.
If you want to learn more about Generative AI interview questions
Integration Challenges
Integrating generative AI into existing healthcare systems presents numerous challenges that require careful consideration to ensure successful implementation. One major challenge is interoperability issues that arise when trying to connect different systems currently used to manage various operations within healthcare organizations.
For instance, if an electronic health record (EHR) system cannot effectively communicate with newly introduced generative AI tools, it may create information silos that hinder clinical decision-making. Additionally, if clinicians lack trust or understanding of these new technologies, they may resist adopting these tools, further complicating integration efforts.
To address these challenges, comprehensive training programs are needed to ensure all relevant personnel can effectively use new technologies and understand their potential benefits. Establishing strong support systems will also be crucial for resolving any issues or concerns during implementation.
The Future of Generative AI in Healthcare
Ongoing Research and Development
As we progress further into the 21st century, technological advancements continue to reshape industries, including healthcare. Ongoing research and development surrounding applications involving generative AI remain critical for maximizing benefits while minimizing risks associated with implementation strategies.
Numerous organizations and universities are actively exploring innovative ways to harness these technologies to improve clinical workflows, enhance patient engagement, and increase operational efficiency. For instance, researchers are developing new algorithms that can better identify and reduce biases to ensure fairness in service delivery.
Additionally, establishing interdisciplinary teams that combine computer scientists with healthcare professionals is essential for driving innovation. This collaboration promotes knowledge sharing and best practices, ultimately leading to more effective solutions that meet the evolving needs of the healthcare industry.
If you want to learn more about Generative AI Salary in India
Regulatory Considerations
With the rapid development of generative AI in healthcare, the need for strong regulatory frameworks becomes increasingly urgent. Policymakers must balance innovation with patient protection to ensure these new technologies are implemented safely and effectively.
For example, regulatory bodies like the Food and Drug Administration (FDA) are beginning to consider how to regulate AI-based medical devices and develop guidelines to ensure their safety and efficacy. At the same time, governments worldwide are working on regulations to protect patient privacy and prevent data misuse.
Moreover, ongoing dialogue with stakeholders—including healthcare providers, technology developers, and patients—is crucial for ensuring that regulatory frameworks reflect the needs and concerns of all parties involved. This collaboration will help build trust and promote the widespread adoption of generative AI in healthcare.
Case Studies in Automation
- Bayer Pharma: This company is improving clinical trial processes by leveraging generative AI to streamline data collection and analysis. This enhances the speed and efficiency of trials, ultimately leading to faster drug development.
- HCA Healthcare: By using generative AI, HCA Healthcare is enhancing documentation and workflow for clinicians. This allows healthcare providers to focus more on patient care rather than paperwork.
- MEDITECH: This organization is making it easier to search and summarize electronic health records (EHRs). With AI-driven solutions, clinicians can quickly access relevant patient information, improving the quality of care provided.
The Impact of Natural Language Processing
Natural Language Processing (NLP) is another form of AI that is reshaping the healthcare landscape. NLP is used in a wide range of health data applications, such as improving patient care through better diagnosis accuracy and streamlining clinical processes. It also provides more personalized services by analyzing patient interactions and feedback.
Applications of NLP in Healthcare
- Improved Diagnosis Accuracy: NLP algorithms can analyze clinical notes and medical literature to assist doctors in making more informed decisions.
- Streamlined Clinical Processes: By automating the extraction of relevant information from unstructured data, NLP helps reduce administrative workload and speeds up clinical workflows.
- Personalized Services: NLP can enhance patient engagement by enabling chatbots and virtual assistants to communicate effectively with patients, providing them with timely information about their health.
Generative AI as a Future Healthcare Technology
Examples of Generative AI in Action
AI in healthcare examples aimed at improving communication include:
- Automated Appointment Systems: These platforms help patients schedule appointments easily without needing to call the clinic.
- Real-Time Health Status Monitoring: This is particularly useful for chronic diseases like diabetes, where continuous monitoring can lead to timely interventions.
- Patient Engagement Solutions: Generative AI can personalize communication with patients based on their health history and preferences.
Future Scope of AI in Healthcare
The future scope of AI in healthcare is multifaceted, with several promising developments on the horizon:
- AI-Driven Imaging and Diagnostics: AI-powered imaging tools can accurately interpret medical images like X-rays and MRIs. Radiologists can leverage AI as a second opinion, improving diagnostic accuracy.
- Enhanced Clinical Decision-Making: With AI, doctors can access immediate and precise data to expedite critical clinical decisions. This leads to quicker diagnoses and treatment plans.
- Cost Savings and Reduced Wait Times: By generating rapid results, AI can help healthcare organizations save costs while reducing patient wait times for both appointments and treatments.
Conclusion
Generative AI and Natural Language Processing are transforming the healthcare landscape by automating routine tasks, enhancing diagnostic accuracy, and improving patient engagement. These technologies streamline clinical workflows, reduce administrative burdens, and provide personalized services that empower both healthcare providers and patients. As the industry continues to adopt these innovations, the potential for improved health outcomes, cost savings, and more efficient care delivery becomes increasingly evident. However, addressing challenges such as data privacy, algorithmic bias, and integration issues will be essential to fully realize the benefits of generative AI in healthcare. The future looks promising as ongoing research and collaboration pave the way for even more advancements in this vital field.
If you want to learn more about Artificial Intelligence Interview questions
Faq's
Generative AI in healthcare refers to artificial intelligence technologies that can create new content or data by learning from existing datasets. This includes generating synthetic patient data, improving diagnostic processes, and developing personalized treatment plans.
Generative AI enhances patient care by providing accurate diagnostics, automating routine tasks, and personalizing communication. This allows healthcare providers to focus more on patient interaction and less on administrative duties.
Applications include clinical decision support systems, drug discovery, patient engagement tools, and administrative task automation such as scheduling appointments and managing records.
Generative AI accelerates drug discovery by analyzing existing chemical and biological data to design new drug candidates efficiently. It can identify potential compounds that meet specific therapeutic criteria.
NLP helps improve patient care by enabling better diagnosis accuracy, streamlining clinical processes, and providing personalized services through chatbots and virtual assistants that understand human language.
Benefits include improved efficiency, enhanced diagnostic accuracy, reduced operational costs, and better patient engagement through personalized communication and automated systems.
Challenges include data privacy concerns, algorithmic bias, integration issues with existing systems, and the need for comprehensive training for healthcare staff.
If training datasets do not accurately represent diverse populations, the resulting algorithms may produce biased outcomes, leading to unequal access to treatment for marginalized groups.
The future scope includes advancements in AI-driven imaging and diagnostics, enhanced clinical decision-making tools, and continued improvements in personalized medicine tailored to individual patient needs.
Successful implementation requires a strategic approach that includes robust training programs for staff, establishing strong regulatory frameworks, and fostering collaboration among stakeholders to ensure that the technology meets the needs of all parties involved.
Want to learn more about Generative AI ?
Join our Generative AI Masters Training Center to gain in-depth knowledge and hands-on experience in generative AI. Learn directly from industry experts through real-time projects and interactive sessions.